The Calculus of Reason - Supervising AI's Mathematical Prowess
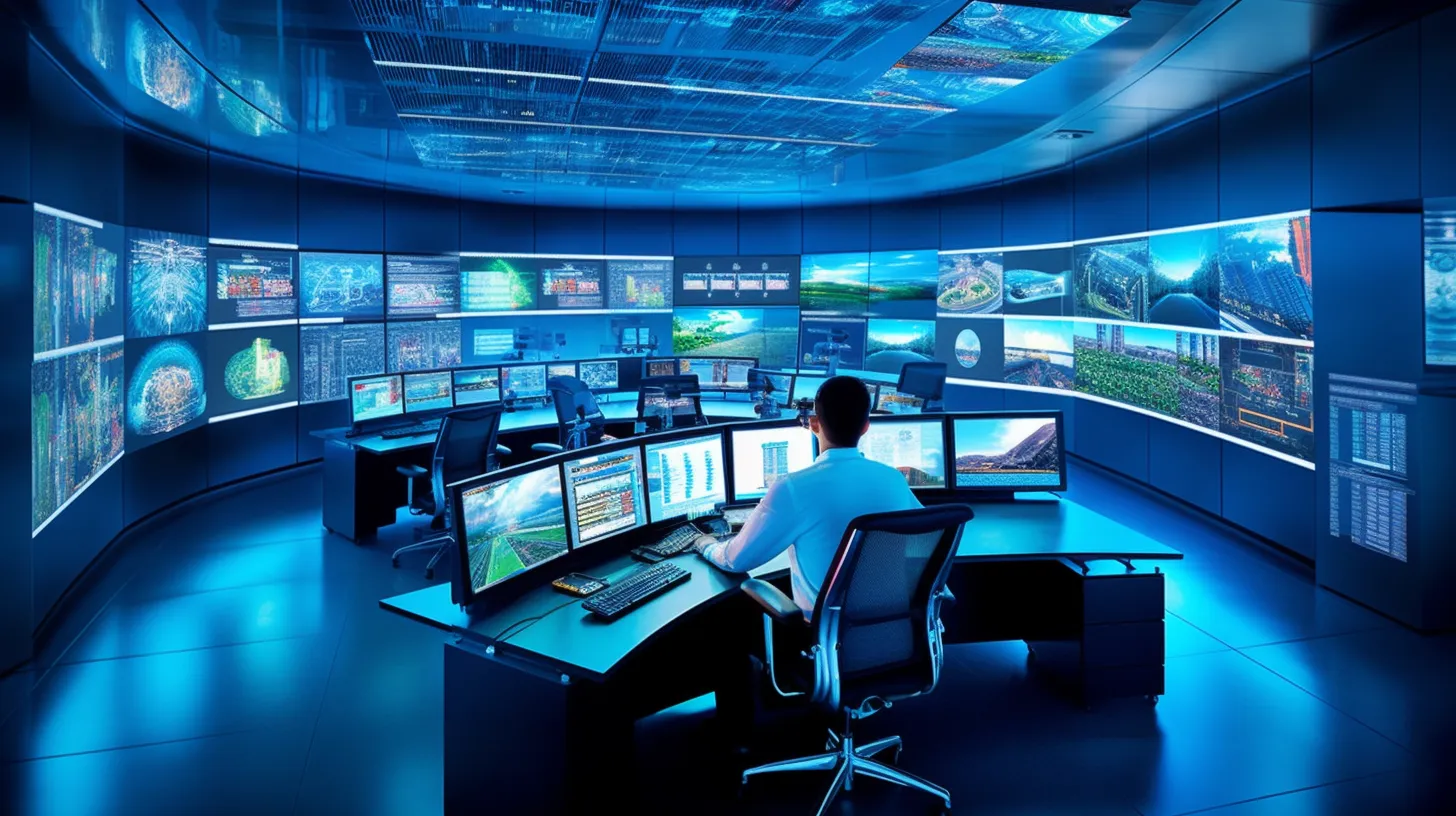
In the realm of artificial intelligence, a quiet revolution is afoot, one that is quietly recalibrating the very calculus of machine reasoning. The revolution is not born of new algorithms or more powerful computing architectures, but of a simple tweak in the way these digital prodigies learn: a concept known as ‘process supervision’.
Not unlike the Socratic method that underpins western pedagogic traditions, process supervision nurtures artificial intelligence by rewarding each step of reasoning, instead of merely the final answer. This approach, as researchers at OpenAI recently demonstrated, has a potent impact on AI’s mathematical prowess. The startling results of their work, however, portend a broader paradigm shift in the way we train and interact with our silicon-based savants.
The MATH dataset, a formidable corpus of mathematical problems, was the chosen arena for OpenAI’s exercise. The AI models were tasked with tackling these problems, equipped with either outcome supervision or process supervision. The latter, quite remarkably, led to better performance - even when judged by outcomes. The implications are profound. In training AI to follow an aligned chain-of-thought, we are closer to creating a breed of AI that not only answers correctly but also reasons in a manner that is human-endorsed.
The beauty of process supervision lies in its inherent alignment with our cognitive processes. It fosters transparency, encouraging models to present a human-approved sequence of thoughts. By contrast, outcome supervision, while simpler, rewards an unaligned process and makes scrutiny of AI reasoning a daunting task.
In the realm of AI safety, there is often a trade-off referred to as the “alignment tax”. This is the cost of ensuring that an AI’s goals align with those of its human operators. It typically leads to reduced performance, which often discourages the adoption of alignment methods. However, process supervision, at least in the math domain, presents an intriguing case where the alignment tax is negative. As we continue to implement process supervision, it could very well lead to AI systems that are not only more performant but also safer.
The performance gap between process-supervised and outcome-supervised models, as observed in the MATH dataset, is a testament to the efficacy of the former approach. The process-supervised model not only performed better across the board, but the gap widened as more solutions were considered for each problem, pointing to its reliability.
The benefits of process supervision are not without caveats. Its success in the mathematical realm is well-demonstrated, but how well it generalizes to other domains remains to be seen. It is not far-fetched to anticipate that process supervision could offer us a method that is both more performant and more aligned than outcome supervision, even in diverse domains.
As we continue to unlock the potential of artificial intelligence, process supervision stands as a beacon of hope, offering us a path to AI systems that are more capable, more transparent, and more aligned with human thought processes. In this journey, we are not only enhancing the capabilities of AI but also fostering a closer alignment with our own cognitive processes, turning the tide in the quest for safe artificial intelligence.
The process of reasoning, be it human or artificial, is a journey, not a destination. As we continue to navigate this exciting landscape, let us remember that in AI, as in education, the journey often matters more than the destination. In a world increasingly shaped by artificial intelligence, the calculus of reason has never been more important.