Retrieval-Augmented Generation: Revolutionizing AI with Real-Time Accuracy and Relevance
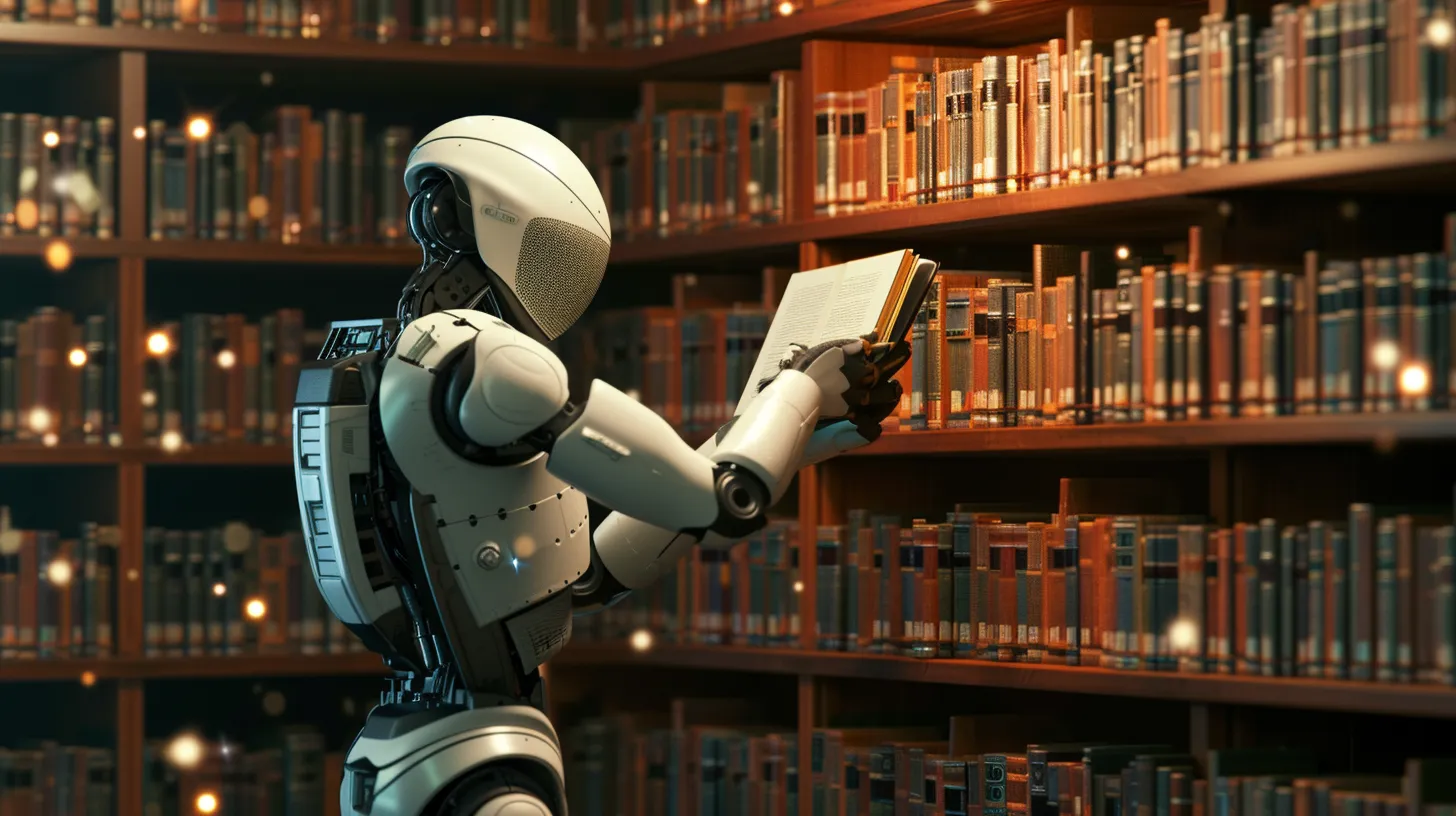
In the rapidly evolving landscape of artificial intelligence (AI), the quest for precision and contextually relevant responses remains a Sisyphean task. Large Language Models (LLMs), the titans of the AI world, have long grappled with the dual challenges of outdated information and the propensity to generate plausible yet erroneous responses, colloquially known as “hallucinations”. This is where Retrieval-Augmented Generation (RAG) emerges as a beacon of hope, a technique poised to revolutionize the way AI interacts with human queries.
The crux of the problem lies in the inherent design of LLMs. Trained on vast corpuses of data, these models are adept at weaving words into coherent, often eloquent, responses. However, their knowledge is frozen at the point of their last training update, rendering them oblivious to the relentless march of time and information. Consequently, when faced with queries demanding up-to-the-minute accuracy or deep dives into niche topics, these models falter, offering responses that are at best outdated and at worst misleading.
Enter RAG, a technique that infuses LLMs with a dynamic edge. At its core, RAG is akin to a digital court clerk, dispatched to fetch relevant facts from a sprawling library of current information. This process involves two critical stages: retrieval and generation. In the retrieval phase, the model scours an external knowledge base – a repository of up-to-date, authoritative information – in response to a user’s query. This step is crucial, as it ensures that the model’s response is grounded in the most current and relevant data available.
The second stage, generation, sees the model synthesizing the retrieved information with its pre-existing knowledge base. This fusion of external data and internal understanding enables the model to craft responses that are not only accurate but also deeply informed by the latest developments in the subject area.
The implications of RAG for the future of AI are profound. By bridging the gap between static knowledge and dynamic information retrieval, RAG-equipped models promise a new era of AI interactions – one marked by enhanced accuracy, relevance, and trustworthiness. In practical terms, this means AI systems that can provide medical professionals with the latest research findings, financial analysts with the most recent market data, or even curious individuals with timely information on unfolding global events.
Moreover, RAG democratizes the process of keeping AI models current. Traditionally, updating an LLM’s knowledge base required a laborious and costly retraining process. RAG circumvents this by allowing models to tap into external databases, effectively keeping their fingers on the pulse of the latest information without the need for constant retraining.
The adoption of RAG also heralds a shift towards more transparent AI. By citing sources and grounding responses in verifiable data, RAG-equipped models offer a level of accountability hitherto unseen in the AI domain. This transparency is not just a boon for user trust; it also represents a stride towards responsible AI, where outputs can be traced back to their origins, and the veracity of AI-generated content can be scrutinized.
As the dawn of the RAG era breaks, the potential applications of this technology stretch as far as the imagination can roam. From customer service bots that can provide real-time, accurate information to virtual assistants that can draw from the latest scientific research, the possibilities are boundless. In a world awash with information, RAG stands as a lighthouse, guiding AI towards a future where accuracy, relevance, and trust are not just ideals, but realities.