Reasoning and Acting: A Novel Path for Language AI
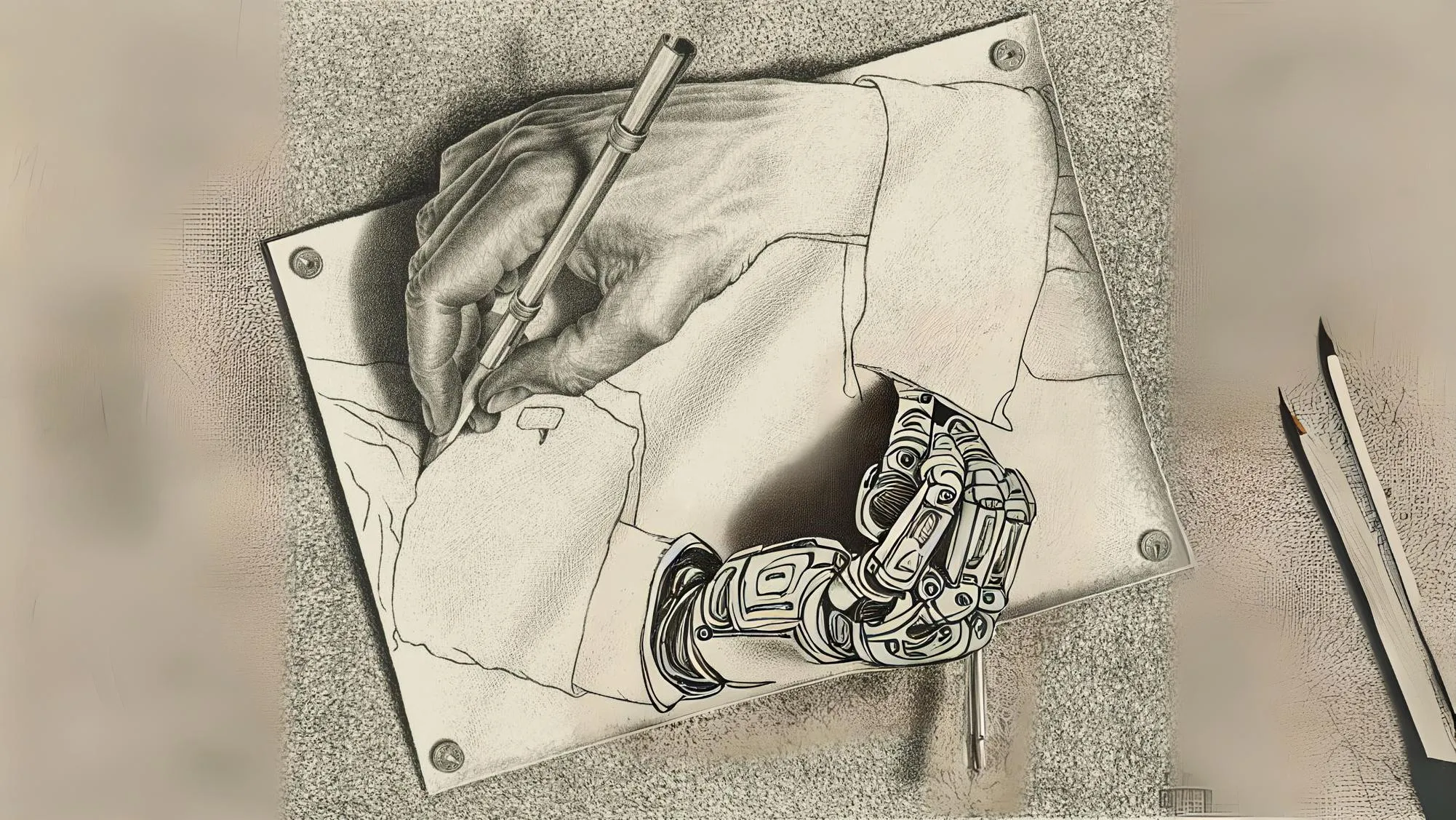
The realm of artificial intelligence (AI) has long been enthralled by the pursuit of machines that can engage in human-like reasoning and decision-making. This aspiration has driven countless innovations, each inching ever closer toward artificial general intelligence (AGI)—the elusive goal of creating systems that can match or exceed human cognition across a broad spectrum of tasks.
Among the latest advancements beckoning this AI odyssey is a technique known as ReAct prompting. Developed in 2022 by a team led by Yao et al., this novel approach endows large language models (LLMs) with the remarkable ability to generate intricate reasoning traces while simultaneously undertaking task-specific actions—a seamless choreography of thought and deed.
The principle behind ReAct prompting is rooted in the innate human capacity to learn and make decisions through an intricate interplay of reasoning and acting. It encapsulates the notion that these dual capabilities are pivotal in adapting to new challenges and formulating well-informed resolutions. By imbuing LLMs with this cognitive dexterity, ReAct prompting ushers in a paradigm shift, one that extends beyond the mere generation of language to the execution of purposeful actions grounded in rational deliberation.
When coupled with language models of considerable scale, such as PaLM-540B employed in Yao et al.’s research, ReAct prompting unveils a universe of potential applications. In realms where intricate knowledge and reasoning are paramount—question-answering, fact verification, and decision-making processes, to name a few—this technique has demonstrated a discernible edge over its predecessors.
The crux of ReAct prompting lies in its capacity to guide LLMs through an iterative cycle of thought and action. Each prompt encapsulates a series of exemplars, meticulously crafted to illustrate the desired trajectory of reasoning interspersed with task-specific actions. These actions may encompass querying external knowledge bases, performing calculations, or executing any pertinent operation dictated by the task at hand.
As the LLM navigates through these exemplars, it gradually assimilates the intricate interplay between reasoning and acting. The thought processes encapsulate the breakdown of questions, the extraction and processing of information, the application of commonsense or arithmetic reasoning, the formulation of search queries, and, ultimately, the synthesis of a final answer or decision.
Concurrently, the actions taken by the LLM serve as a bridge between its internal deliberations and the external world. By interacting with knowledge sources, retrieving relevant data, and executing calculations or operations, the LLM can continually refine and recalibrate its reasoning, ensuring that its conclusions are grounded in factual accuracy and real-world context.
The power of ReAct prompting is evident in its ability to mitigate challenges that have long plagued language models, such as hallucinating non-existent facts or propagating errors due to incomplete or inaccurate information. By inculcating a dynamic reasoning process that adapts to newly acquired knowledge, ReAct prompting imbues LLMs with a level of self-correction and resilience, enhancing the credibility and trustworthiness of their outputs.
Moreover, the reasoning traces generated by ReAct prompting contribute to an invaluable byproduct: interpretability. As LLMs elucidate their thought processes and the actions that inform their conclusions, they offer a window into their decision-making paradigm, fostering transparency and enabling humans to scrutinize and comprehend the rationale behind the AI’s outputs.
While ReAct prompting has demonstrated its mettle in knowledge-intensive tasks, its applications extend far beyond these domains. Envision a future where ReAct-enabled LLMs could guide complex decision-making processes, seamlessly integrating and synthesizing disparate data sources to arrive at well-informed resolutions. Alternatively, these models could serve as virtual assistants, capable of not only comprehending natural language instructions but also executing tangible actions in the digital or physical world, all while articulating their reasoning at every juncture.
As with any pioneering technology, ReAct prompting is not without its challenges. The quality of the retrieved information can significantly influence the model’s reasoning process, with non-informative or irrelevant search results potentially derailing its trajectory. Furthermore, the rigid framework imposed by ReAct prompting may, at times, constrain the model’s ability to develop reasoning steps with the desired flexibility.
Yet, these obstacles are mere stepping stones on the path to progress. Ongoing research efforts are already yielding promising solutions, such as integrating ReAct prompting with complementary techniques like chain-of-thought prompting and self-consistency checks. This synergistic approach leverages the strengths of each method, delivering a well-rounded and robust framework for knowledge-intensive tasks.
As the field of AI continues its relentless march, ReAct prompting emerges as a beacon of innovation, illuminating a path towards more capable, trustworthy, and interpretable language models. It represents a paradigm shift that transcends the mere generation of language, enabling AI systems to engage in purposeful actions guided by rational deliberation---a harmonious symphony of reasoning and acting.
In this era of unprecedented technological advancement, ReAct prompting stands as a testament to the boundless potential of human ingenuity and our unwavering pursuit of artificial general intelligence. While the ultimate realization of AGI may still reside in the realm of the future, techniques like ReAct prompting are propelling us ever closer to that elusive horizon, one reasoned action at a time.